Building enzymes with AI
Briefs
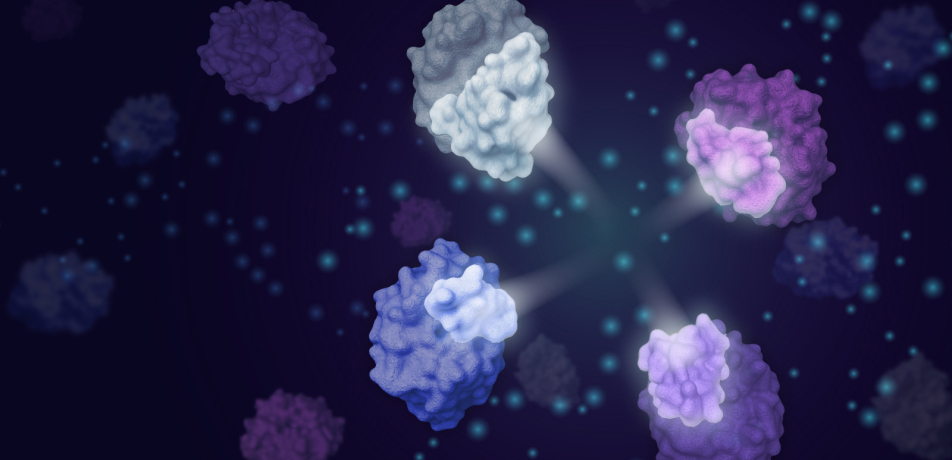
An illustration showing four modular enzyme fragments the CADENZ method can combine to generate active enzymes at a previously unseen rate. (Image from the Fleishman lab)
Tailored appropriately, enzymes have the potential to transform the chemical industry by providing green alternatives for a range of processes. Enzymes act as biological catalysts—and with the help of molecular engineering, they can shift naturally occurring reactions into turbo mode. Examples include enzymes that enable nonpolluting drug manufacture, or those that break down pollutants, sewage, and agricultural waste safely, and then turn the resulting products into biofuel or animal feed.
A new study, led by Prof. Sarel Fleishman in the Department of Biomolecular Sciences and published recently in Science, brings this vision closer to reality. The Fleishman group unveiled an artificial intelligence/computational method for designing thousands of diverse and active enzymes with unprecedented efficiency, by assembling them from engineered modular building blocks.
Biochemists typically design new enzymes by randomly tweaking the DNA of naturally existing ones and screening the resultant variants for desired activities—an extremely time-consuming process that often gets “stuck” in suboptimal solutions. Taking inspiration from how the immune system generates billions of different antibodies in response to novel pathogens, the Fleishman group decided to generate large numbers of diverse enzymes by breaking down natural ones into constituent fragments that could then be designed and recombined in various ways.
Rosalie Lipsh-Sokolik, a PhD student who led the study in the Fleishman lab, experimented with a family of enzymes that breaks down xylan, a common component of plant cell walls. “If we manage to boost the activity of these enzymes, they might be used for breaking down plant compounds such as xylan and cellulose into sugars, which in turn can help generate biofuels,” she explains. “Instead of disposing of agricultural waste, we should be able to turn it into an energy source.”
Lipsh-Sokolik developed an algorithm that broke down each variant of xylan, splitting the enzymes into fragments and then introducing dozens of mutations into those pieces—all in ways that maximized the potential compatibility of the different bits. The algorithm then assembled fragments into different combinations and selected a million enzyme sequences that were deemed stable.
From there, the team synthesized one million actual enzymes from these computer models and tested them in the lab. To their amazement, 3,000 were confirmed to be active. And while a 0.3% rate may not seem high, protein design studies typically generate only about a dozen active enzymes at best.
Using machine-learning tools, the team examined about 100 features that characterize enzymes and used the 10 most promising ones to create an “activity predictor” that could feed back into the algorithm. The outcome? A tenfold increase in success rate over the initial experiment, and an unparalleled feat in the history of protein design: The team managed, in a single experiment, to design more potentially active enzymes than standard methods could produce in a decade.
Moreover, the active enzymes were exceptionally diverse in terms of both sequence and structure, which suggests they may perform a variety of new functions. The new method, which the scientists call CADENZ—short for Combinatorial Assembly and Design of Enzymes—can, theoretically, be applied to any family of proteins. The Fleishman group is now exploring how to apply this method to generate new, improved antibodies or variants of the fluorescent proteins used as labels in biology.
“Protein engineering is becoming a central part of the economy and public health: Industrial enzymes are proteins; antibodies and vaccines are also proteins,” says Prof. Fleishman. “We need to be able to optimize them and to generate new ones in a robust and reliable way.”
Sarel Fleishman is supported by:
- Artificial Intelligence for Smart Materials Research Fund, in Memory of Dr. Uriel Arnon
- Schwartz Reisman Collaborative Science Program
- Dr. Barry Sherman Institute for Medicinal Chemistry
- Sam (Ousher) Switzer & Children