Saving lives with AI
Artificial intelligence is enabling scientists to tackle pressing questions in biomedical research
Features

From multiple myeloma to nerve repair, Weizmann Institute scientists are using artificial intelligence (AI) and big data tools to reach new discoveries related to human health and disease. In a modern twist on the scientific method—the hypothesis-driven data analysis that has traditionally been the foundation of knowledge acquisition—some of today’s most important biomedical insights are emerging from datasets so huge that they can only be analyzed with the help of AI technologies.
The historical foundation of AI is machine learning, an approach in which a computer, exposed to a large amount of training data, eventually gains the ability to recognize and categorize data it has not seen before, without being explicitly programmed to do so. More advanced AI protocols, like “deep learning” approaches based on artificial neural networks, allow computerized systems to reveal patterns in complex data, make independent decisions, and even identify novel questions worth pondering. This AI-empowered path to discovery is not science fiction; it is already transforming the world of scientific research.
The Artificial Intelligence Enterprise for Scientific Discovery, a flagship initiative of the Weizmann Institute, is leveraging this paradigm shift in the world of research, by developing and implementing AI tools for accelerating progress across all areas of the exact sciences. Building on the Institute’s preeminence in computer science and neurobiology, this project will increase Weizmann scientists’ ability to interpret the findings emerging from today’s data-heavy research disciplines.
Some of the most exciting AI-related developments taking place in life science labs at the Weizmann Institute are presented below.
Machine learning for multiple myeloma
Prof. Ido Amit of the Department of Immunology has identified several pathways that were active in people with resistant multiple myeloma, but not in healthy donors or in patients whose myeloma had responded to treatment. To do so, he used an AI protocol called machine learning, as well as single-cell analysis, as a way to characterize various gene expression profiles associated with this type of blood cancer. This AI-based approach also allowed Prof. Amit to identify a novel and potent drug for the treatment of resistant patients, something that may bring new hope to multiple myeloma sufferers worldwide.
Post-injury nerve repair
Prof. Ronen Basri, of the Department of Computer Science and Applied Mathematics, studies various aspects of computer vision and machine learning. His work has already had practical, biomedical impact: a computer vision system based on an algorithm developed by Prof. Basri and developed by staff scientist Dr. Meirav Galun scans neurons in a petri dish, providing automated and highly accurate accounting of how the axons of nerve cells re-grow after injury. This technique can quantify the overall growth of complex assemblies of multiple neurons, providing neuroscientists with data that may someday contribute to clinical strategies for nerve repair.
Who benefits from anti-depressants?
Selective serotonin reuptake inhibitors (SSRIs) are first-line treatment for both depression and anxiety. However, it has been reported that 30%–40% of all patients fail to respond. Moreover, SSRI use for children and adolescents is frequently associated with serious adverse events, including increased suicidal behavior. Working together with Dr. Maya Amitai, a senior physician at Schneider Children’s Medical Center and a PhD student in his lab, Prof. Alon Chen — President of the Weizmann Institute and a faculty member in the Department of Brain Sciences — is using machine learning to identify biological markers that can help determine which patients are most likely to benefit from SSRI treatment. Such markers can also help clinicians avoid the use of SSRIs for those children and adolescents prone to developing suicidal behavior.
Faster, more accurate disease diagnosis
AI can improve clinicians’ ability to use medical imaging technologies to assess and diagnose diseases. Prof. Yonina Eldar, Director of the Weizmann Institute’s Manya Igel Centre for Biomedical Engineering and Signal Processing, along with Dr. Keren Peri-Hanania, a physician who is the Center’s clinical manager, are using advanced AI approaches, such as model-based deep learning, to improve the efficacy of such technologies as X-ray and ultrasound for the diagnosis of conditions like cancer, inflammatory bowel diseases and Covid-19. Using AI to identify imaging abnormalities, to generate high-resolution images, to combine different imaging modalities, and to guide inexperienced technicians in the acquisition of ultrasound images, Prof. Eldar and her clinical colleagues seek to reduce errors and advance early disease detection, something that will lead to improved treatment outcomes.
Cutting through noisy medical images
High resolution imaging technologies―from medical MRI to the electron microscopes used in scientific research―can produce “noisy” data that make it difficult to focus on what’s really important. Prof. Yaron Lipman is an applied mathematician who is adapting classical AI approaches to create tools that interpret noisy and unstructured data, and help direct the work of technicians charged with acquiring and analyzing images. His AI protocols, which have been successfully applied to everything from medical ultrasound to experiments in particle physics, provides users of advanced technology platforms with automated identification of significant data in noisy environments, and guides them toward accurate discoveries or clinical diagnoses that might never have been achieved without AI support.
A closer, computerized, look at pancreatic tumors
Recent research by Prof. Michal Neeman of the Department of Biological Regulation demonstrated the use of computer vision—an important factor in many AI technologies—for the direct visualization of pancreatic tumors. She used “intravital imaging”, an approach in which cells of a live animal are examined through an imaging window surgically implanted into the animal tissue. This automated imaging yielded quantifiable markers that distinguish the normal pancreas from abnormal tumor vasculature during pancreatic tumor progression.
Toward personalized lung cancer treatment
One of the challenges of clinical oncology is predicting which cancer patients will respond best to specific drugs. Using artificial intelligence, Prof. Moshe Oren of the Department of Molecular Cell Biology has advanced our ability to match patients with appropriate treatments. AI-assisted analysis of data from patients with non-small cell lung cancer revealed gene expression profiles associated with differential response to particular anticancer compounds. His findings may eventually contribute to better stratification of lung cancer patients, and the assignment of personalized anticancer therapies.
Sting operation in malaria
In a study of malarial infection, Dr. Neta Regev-Rudzki and Prof. Michal Sharon, both of the Department of Biomolecular Sciences, discovered an extraordinary strategy by which the malaria parasite primes the body’s red blood cells, clearing the way for a successful invasion. Working with Dr. Irit Rosenhek-Goldian and Dr. Sidney R. Cohen of the Chemical Research Support Department and Dr. Ido Azuri from the Bioinformatics Unit, Department of Life Sciences Core Facilities, the scientists developed a machine-learning algorithm that distinguishes red blood cells that had been altered by the EV-20S proteasome—a cellular machinery that breaks down proteins—from those that had not. They showed that EV-20S, secreted by the malaria parasite, makes red blood cells an “accessory” to the invasion by altering membrane stiffness in a way that facilitates malarial growth.
Identifying inflammatory diseases before they begin
Prof. Irit Sagi of the Department of Biological Regulation studies the cellular microenvironment, which includes the extracellular matrix (ECM), a collection of secreted molecules that provide both chemical and mechanical stimuli affecting cellular behavior. While the main role of the ECM is to surround and protect cells, when misled by confusing signals, it can also promote tumor development and accelerate inflammation. In recent work on the inflammatory signaling process, Prof. Sagi has been exploring the use of AI tools to characterize pre-symptomatic tissue remodeling processes, something that might enable very early diagnosis, or even the prevention of inflammatory disease.
Predicting risk of gestational diabetes
Prof. Eran Segal, a member of both the Department of Computer Science and Applied Mathematics and the Department of Molecular Cell Biology, has designed an algorithm that can predict the risk of gestational diabetes even before pregnancy. This advance, based on machine learning algorithms that revealed clinically significant patterns in population-wide data from Clalit, Israel’s largest HMO, may one day allow doctors to prevent gestational diabetes in specific patients, by prescribing lifestyle interventions for reducing high blood sugar.
Earlier risk assessment and diagnosis for AML
A collaboration between two Weizmann scientists has led to an AI-based strategy for the early diagnosis of acute myeloid leukemia (AML). Prof. Amos Tanay, of the Department of Biological Regulation and the Department of Computer Science and Applied Mathematics, together with Dr. Liran Shlush of the Department of Immunology, used a combination of deep sequencing and machine learning to identify a distinct gene mutation profile that accurately predicted which patients would develop AML, and which would live to a healthy old age, without developing the disease. The scientists’ approach could potentially be used to identify pre-AML risk many years prior to disease onset.
Prof. Ido Amit is supported by the Eden and Steven Romick Professorial Chair, the Adelis Foundation, Miel de Botton, the Elsie and Marvin Dekelboum Family Foundation, the Joel and Mady Dukler Fund for Cancer Research, the Dwek Institute for Cancer Therapy Research, the Ben B. and Joyce E. Eisenberg Foundation, the Belle S. and Irving E. Meller Center for the Biology of Aging, the Moross Integrated Cancer Center, Richard and Jacqui Scheinberg and Garvan Weizmann Partnership donors, the Thompson Family Foundation Alzheimer’s Disease Research Fund, the Vainboim Family, and the Wolfson Family Charitable Trust and Wolfson Foundation.
Prof. Ronen Basri is supported by the Elaine and Bram Goldsmith Professorial Chair of Applied Mathematics, the Arthur and Rochelle Belfer Institute of Mathematics and Computer Science, and the Ida Kohen Center for Mathematics Research.
Prof. Alon Chen is supported by the Vera and John Schwartz Professorial Chair in Neurobiology, the Licht Family, and the Ruhman Family Laboratory for Research in the Neurobiology of Stress.
Prof. Yonina Eldar is supported by the Dorothy and Patrick Gorman Professorial Chair, the Benoziyo Endowment Fund for the Advancement of Science, the Brenden-Mann Women’s Innovation Impact Fund, Miel de Botton, Jean and Terry de Gunzburg, Manya Igel Centre for Biomedical Engineering and Signal Processing, Henry Chanoch Krenter Institute for Biomedical Imaging and Genomics, and the Sagol Weizmann-MIT Bridge Program.
Prof. Yaron Lipman is supported by the Henri Gutwirth Prize.
Prof. Michal Neeman is supported by the Helen and Morris Mauerberger Professorial Chair in Biological Sciences, the Benoziyo Endowment Fund for the Advancement of Science, the Henry Chanoch Krenter Institute for Biomedical Imaging and Genomics and Fondazione Henry Krenter, the Sagol Institute for Longevity Research, the David and Fela Shapell Family Institute for Preclinical Studies, and the Wolfson Family Charitable Trust and Wolfson Foundation.
Prof. Moshe Oren is supported by the Andre Lwoff Professorial Chair in Molecular Biology, Dr. Miriam and Sheldon G. Adelson Medical Research Foundation, the Moross Integrated Cancer Center, the Robert Bosch Foundation, and Anat and Amnon Shashua.
Dr. Neta Regev-Rudzki is supported by the Benoziyo Endowment Fund for the Advancement of Science and Instituto Serrapilheira.
Prof. Irit Sagi is supported by the Maurizio Pontecorvo Professorial Chair, the Azrieli Foundation, and Madame Olga Klein – Astrachan.
Prof. Eran Segal is supported by the Louis H. Sackin Research Fellow Chair in Computer Science, the Crown Human Genome Center, Miel de Botton, and White Rose International Foundation.
Prof. Michal Sharon is supported by the Aharon and Ephraim Katzir Memorial Professorial Chair in Translational Research, the Benoziyo Fund for the Advancement of Science, the Moross Integrated Cancer Center, and the Sagol Institute for Longevity Research.
Dr. Liran Shlush is supported by the Ruth and Louis Leland Career Development Chair, the Appplebaum Foundation Research Fellow Chair, the Applebaum Foundation, Inc., the Moross Integrated Cancer Center, Rising Tide Foundation, the Sagol Institute for Longevity Research, the Swiss Society Institute for Cancer Prevention Research, and the Morris and Ruth Wagner and Marek Sutkiewicz Laboratory for Cancer Research.
Prof. Amos Tanay is supported by Barry and Janet Lang, Ilana and Pascal Mantoux Institute for Bioinformatics, and the Wolfson Family Charitable Trust & Wolfson Foundation.

Prof. Ronen Basri

Prof. Yonina Eldar

Prof. Yaron Lipman

Dr. Neta Regev-Rudzki
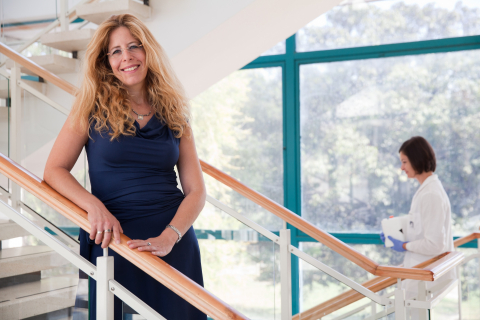
Prof. Michal Sharon